Transforming Customer Data into Strategic Advantage
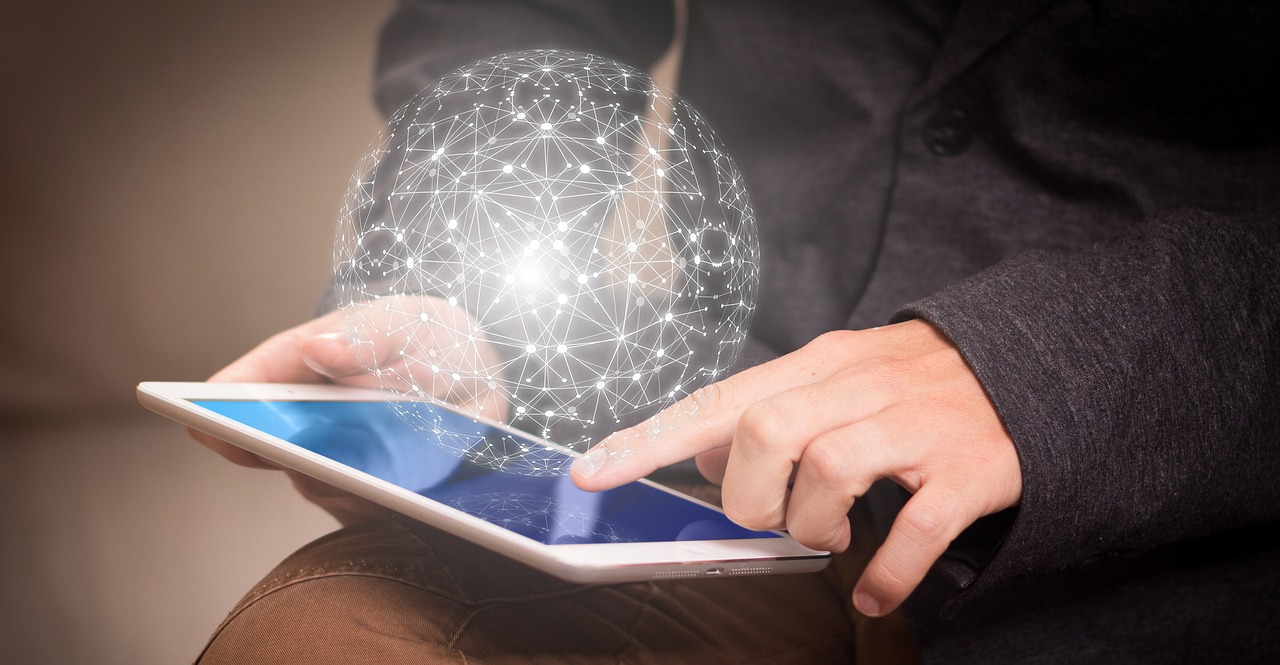
We all collect customer data, but how many of us actually use it effectively? There’s a surprisingly wide gap between collecting data and taking meaningful action on it. This guide explores practical ways to bridge that divide by building a solid data foundation, making sense of customer behaviour patterns, and creating personalised experiences that truly connect with your customers. We’ll show you how to break down those frustrating organisational silos and make customer insights accessible to everyone who needs them—without forcing them to get a PhD in data science first.
What’s the real difference between companies that just pile up customer information and those that gain genuine competitive advantage through data? It’s not about how much data you gather—it’s about what you do with it. Throughout this guide, we’ll tackle the common challenges that keep organisations from making the most of their customer data, while offering practical frameworks to turn raw information into strategies that boost satisfaction, build loyalty, and drive growth. The key question isn’t whether you have enough data—it’s whether you’re asking the right questions of the data you already have.
Building Your Customer Data Foundation
Identifying Essential Data Sources for Comprehensive Customer Understanding
Let’s face it—meaningful customer analysis goes far beyond basic demographics. Yes, age, location, and income give you context, but the gold mine is in behavioural and transactional data that show what customers actually do rather than what they claim they’ll do. Forward-thinking companies are now pulling together multiple data streams: purchase history, browsing behaviour, support conversations, social media engagement, and even IoT device usage to build multidimensional customer profiles.
Transaction data is particularly valuable because it provides hard evidence of customer preferences and spending patterns. Just as important are interaction metrics that show how customers engage with your digital properties—how long they spend on specific pages, where they abandon carts, and what content they consume. When you use data and technology thoughtfully, you can create experiences that genuinely connect with individual customers rather than broad segments. This kind of personalisation transforms your relationship from transactional to truly consultative.
The most sophisticated customer data strategies also tap into external data sources that provide contextual understanding. These might include industry benchmarks, economic indicators, seasonal trends, and competitor positioning that help explain changes in customer behaviour that your internal data alone can’t illuminate. When combined with your own customer information, these external signals create a much richer understanding of why customers make the decisions they do.
Creating a Unified Customer Data Platform for Holistic Analysis
Data fragmentation is one of the biggest barriers to really understanding your customers. When customer information is scattered across disconnected systems—CRM platforms, marketing automation tools, support ticketing systems, billing software—it’s nearly impossible to develop a coherent view of the customer journey. Implementing a unified customer data platform (CDP) addresses this challenge by creating a single source of truth that brings together information from all these scattered sources.
The best CDPs do more than just aggregate data—they provide identity resolution capabilities that connect customer activities across devices and channels. This creates a persistent customer profile that maintains continuity even as people move between your website, mobile app, physical store, and call centre. With the right implementation, your CDP should provide both historical context and real-time updates, helping you understand not just who your customers were, but who they’re becoming.
Customer analytics helps you identify and examine patterns in customer behaviour to uncover opportunities for improving business outcomes across your entire organisation. By centralising this capability, you can democratise access to insights while maintaining appropriate governance controls. The right CDP implementation should balance technical sophistication with usability, ensuring that customer insights are accessible to business users without requiring them to become data scientists.
Establishing Data Quality Protocols for Insight Accuracy
The value of your customer insights depends entirely on the quality of your data. You need to implement rigorous data quality protocols that address completeness, accuracy, consistency, timeliness, and relevance. This starts with establishing clear data governance policies that define ownership, access rights, and maintenance responsibilities for customer information across your organisation.
Automate data validation processes wherever possible, with systems that flag anomalies, duplicates, and inconsistencies before they contaminate your analysis. Regular data audits should assess both technical quality metrics and business relevance factors—ensuring that you’re not only collecting accurate information but information that actually matters to your strategic objectives. Document and communicate these protocols across your organisation to create accountability for data quality at every touchpoint.
Effective data quality management requires both technology and human oversight. While automation can handle many validation tasks, you need subject matter experts to regularly review insights to ensure they align with business realities. This collaborative approach helps identify situations where technically “correct” data leads to misleading conclusions, preventing costly strategic missteps based on flawed analysis.
Balancing Quantitative Metrics with Qualitative Customer Feedback
Numbers tell an important part of the customer story, but rarely the whole story. If you rely exclusively on quantitative metrics, you’ll miss the emotional and contextual factors that drive customer decisions. A comprehensive customer data foundation must integrate structured quantitative data with unstructured qualitative feedback from sources like:
- Voice of customer surveys and NPS responses
- Social media comments and sentiment analysis
- Customer support transcripts and call recordings
- User testing sessions and usability feedback
- Sales call notes and customer interviews
Integrating qualitative and quantitative insights creates a more nuanced understanding of customer behaviour. Customer behaviour analysis provides valuable insights into satisfaction levels, loyalty patterns, and churn risks that purely quantitative approaches might miss. This balanced approach helps you understand not just what customers are doing, but why they’re doing it—transforming raw data into actionable intelligence that can drive your business strategy.
Text analytics and natural language processing tools now make it possible to analyse qualitative feedback at scale, identifying patterns and themes across thousands of customer comments. When combined with traditional metrics, these technologies help you identify the root causes behind customer behaviours, creating opportunities for more meaningful engagement and service improvements.
Transforming Analytics into Personalisation Opportunities
Moving Beyond Basic Personalisation to Individualised Experiences
Basic personalisation—inserting a customer’s name into communications or recommending products based on past purchases—no longer differentiates brands in today’s competitive landscape. Leading organisations are now implementing individualisation strategies that adapt entire experiences to each customer’s unique context, preferences, and needs. This shift from segment-based personalisation to true individualisation requires more sophisticated data models and delivery systems, but delivers substantially higher engagement and conversion rates.
Individualisation considers not just who the customer is, but their current context—device, location, time of day, recent interactions—to deliver precisely the right experience at the right moment. This approach recognises that the same customer may have different needs and preferences depending on whether they’re researching on a desktop during work hours or browsing on mobile during their evening commute. By adapting to these contextual factors, you can create more relevant experiences that feel genuinely helpful rather than simply personalised.
The most advanced individualisation approaches incorporate real-time behavioural signals that allow experiences to adapt within a single session. When a customer exhibits browsing patterns that indicate specific interests or intent, content, offers, and navigation can immediately adjust to better serve their needs. This dynamic responsiveness creates the impression of an intuitive experience that anticipates customer needs before they’re explicitly expressed.
Leveraging Predictive Analytics to Anticipate Customer Needs
Predictive analytics transforms customer data from a historical record into a forward-looking strategic asset. By identifying patterns in past behaviour that correlate with future actions, you can anticipate customer needs and preferences with remarkable accuracy. These predictions enable proactive engagement that positions offerings at precisely the moment when customers are most receptive, creating both higher conversion rates and stronger customer satisfaction.
Need anticipation models analyse behavioural sequences to identify when customers are likely to require specific products, services, or support interventions. For example, usage patterns might indicate when a customer is approaching a natural upgrade point, or engagement signals might reveal when a customer is struggling with a particular feature. By recognising these patterns, you can initiate relevant outreach before customers explicitly request assistance, creating the impression of exceptional service and attention.
Intent prediction models go beyond identifying needs to forecast specific actions customers are likely to take. By analysing the behavioural patterns that typically precede purchases, cancellations, or other high-value actions, you can identify customers with high conversion or churn propensity. These predictions enable more precise targeting for acquisition and retention efforts, focusing resources where they’ll deliver the greatest impact.
Creating Dynamic Content Frameworks That Respond to Behavioural Signals
Static content strategies simply can’t deliver truly personalised experiences in a world where customer needs and interests change continuously. Dynamic content frameworks provide the flexibility to assemble individualised experiences from modular components based on customer data and behavioural signals. This approach enables you to create seemingly infinite variations from a manageable content library, ensuring relevance without unmanageable production requirements.
Component-based content architectures separate content elements—headlines, images, product descriptions, offers—into discrete modules that can be independently selected based on customer attributes and behaviours. This modular approach allows for combinatorial personalisation that can generate thousands of unique experience variations from a relatively small set of base components. When implemented effectively, this strategy balances personalisation sophistication with operational efficiency.
Decision rules and machine learning algorithms determine which content components to display for each customer based on their profile and behavioural patterns. These selection mechanisms consider multiple factors—from explicit preferences to implicit behavioural signals—to assemble the optimal experience for each interaction. As customer analytics helps identify and examine patterns in behaviour, these insights can be translated directly into more relevant content experiences that improve business outcomes.
Measuring Personalisation Impact Through Controlled Experimentation
You can’t just assume personalisation effectiveness—you need to rigorously measure it through controlled experimentation. A/B and multivariate testing methodologies allow you to isolate the impact of personalisation strategies by comparing performance against control groups that receive standard experiences. This experimental approach provides clear evidence of personalisation ROI while identifying which approaches deliver the greatest value.
Incrementality testing is a particularly valuable methodology for measuring personalisation impact. By withholding personalised experiences from a randomly selected control group, you can measure the true lift provided by personalisation efforts rather than simply observing overall performance. This approach helps distinguish between actions customers would have taken anyway and those genuinely influenced by personalisation strategies.
Long-term impact assessment extends beyond immediate conversion metrics to evaluate how personalisation affects customer lifetime value, retention rates, and advocacy behaviours. The most sophisticated measurement approaches track cohorts over time to identify how personalised experiences influence customer relationships throughout the lifecycle. This longitudinal view helps you balance short-term conversion optimisation with long-term relationship development, creating sustainable personalisation strategies that build lasting customer value.
Ethical Considerations in Customer Data Analysis
Navigating Privacy Regulations While Maintaining Analytical Depth
The regulatory landscape for customer data continues to evolve rapidly, with frameworks like GDPR, CCPA, and emerging state-level privacy laws creating complex compliance requirements. You need to develop sophisticated approaches that respect both regulatory boundaries and customer privacy expectations while still enabling meaningful analysis. This balance requires thoughtful data governance that goes beyond minimum compliance to establish sustainable data practices.
Data minimisation principles help you collect only information with clear analytical value, reducing both regulatory risk and storage costs. By critically evaluating each data element against its potential business value, you can focus collection efforts on high-impact information while eliminating unnecessary data capture. This selective approach simplifies compliance while often improving analytical focus by reducing noise in customer datasets.
Anonymisation and pseudonymisation techniques provide mechanisms for conducting powerful analytics while protecting individual privacy. By separating identifying information from behavioural and transactional data, you can derive valuable insights without compromising customer identities. These approaches, when properly implemented, enable sophisticated pattern analysis while maintaining regulatory compliance and customer trust.
Creating Transparent Data Policies That Build Customer Trust
Transparency represents the foundation of ethical customer data practices. You must clearly communicate what information you collect, how you use it, and what benefits customers receive in exchange. This transparency builds trust while giving customers meaningful control over their information—creating more sustainable relationships than opaque data practices that prioritise short-term analytical advantages.
Privacy policies should be written in accessible language that helps customers understand actual data practices rather than simply providing legal protection. The most effective policies explain not just what data is collected, but why it matters and how it benefits the customer experience. This explanatory approach helps customers make informed decisions about data sharing while demonstrating your respect for their agency.
Preference management systems give customers granular control over how their data is used, allowing them to selectively opt into specific data uses based on their personal privacy preferences. These systems recognise that privacy tolerance varies widely among individuals—some customers willingly share extensive information in exchange for personalised experiences, while others prioritise privacy over personalisation. By providing choice rather than one-size-fits-all approaches, you respect this diversity while maximising data availability from willing participants.
Balancing Personalisation with Privacy Concerns
The tension between personalisation and privacy represents one of the central challenges in customer analytics. Customers increasingly expect tailored experiences but remain concerned about how their data is used to create them. You must navigate this paradox by developing personalisation approaches that deliver value while respecting privacy boundaries and maintaining customer comfort.
Value exchange clarity helps customers understand the specific benefits they receive in exchange for sharing their information. When you explicitly connect data sharing to experience improvements—showing how providing certain information enables specific personalisation features—customers can make informed decisions about the tradeoffs involved. This transparent approach builds trust while increasing willingness to share information when the benefits are clear.
Progressive personalisation models start with minimal data requirements and gradually increase personalisation sophistication as customers demonstrate comfort through continued engagement. This incremental approach respects privacy concerns while allowing the relationship to deepen naturally over time. By earning the right to more extensive personalisation through consistent value delivery, you build sustainable data relationships rather than demanding extensive information upfront.
Establishing Ethical Frameworks for Responsible Data Usage
Beyond legal compliance, you need ethical frameworks that guide decisions about appropriate data usage. These frameworks should establish principles that reflect your organisational values while providing practical guidance for teams working with customer information. When properly developed and implemented, ethical data frameworks help you navigate ambiguous situations where regulations provide limited direction.
Fairness principles ensure that your data practices don’t create or amplify biases that disadvantage specific customer groups. Regular bias audits should examine how algorithms and personalisation systems affect different customer segments, identifying and addressing any disparate impacts before they create customer harm or regulatory issues.
From Data Collection to Strategic Advantage
The difference between organisations that merely accumulate customer data and those that transform it into competitive advantage isn’t found in the volume of information gathered, but in how effectively they interpret and activate it. By establishing robust data foundations, conducting sophisticated behavioural analysis, implementing meaningful personalisation, and democratising insights across departments, you can bridge the gap between raw information and strategic action. When supported by ethical frameworks that balance analytical depth with privacy considerations, these approaches create a virtuous cycle where customer understanding drives business results while strengthening relationships.
The journey from data collection to customer insight isn’t primarily a technical challenge—it’s a strategic one. It requires asking the right questions of your existing data rather than simply gathering more information. When you master this discipline, you don’t just understand your customers better; you anticipate their needs, personalise their experiences, and build relationships that competitors can’t easily replicate. In today’s data-rich environment, your competitive advantage doesn’t depend on what you know about your customers—it depends on what you do with that knowledge before someone else does.